Restructuring data teams that are ready to scale: 5 learnings from BlablaCar
Written by Emmanuel Martin-Chave, an expert in Tech and Data, who grew the Data team 8x to 40 people, moved to the cloud and adopted leading-edge technologies in his 7 years at BlaBlacar. He also co-founded and currently champions the Modern Data Network community in France.
In today's interconnected world, data has become a powerful driving force behind innovation and growth. Companies that harness the potential of data hold a competitive edge, and one such company at the forefront is BlaBlaCar. As a pioneer in the carpooling industry, BlaBlaCar has revolutionized the way people travel and the way it leverages data plays a critical role in its business strategy.
In this article, we delve into the fascinating world of BlaBlaCar's data team strategy, exploring how we restructured our teams in order to scale.
Now: What BlaBlaCar looks like
BlaBlaCar’s Data department sits within the Engineering organization. It is an entity of around forty people, divided into 6 teams: 5 multidisciplinary squads, and a platform-oriented team.
Many data professions are represented in this entity: Data Analysts, Data Scientists, Software Engineers and Data Engineers. Thus, they have full autonomy to carry out end-to-end projects.

Before: What BlaBlaCar looked like then
This transformation story starts in 2021. At the time, the Data department was organized by technological layers: a Data Engineering team, a Data Science team, a Data Analytics one, etc.
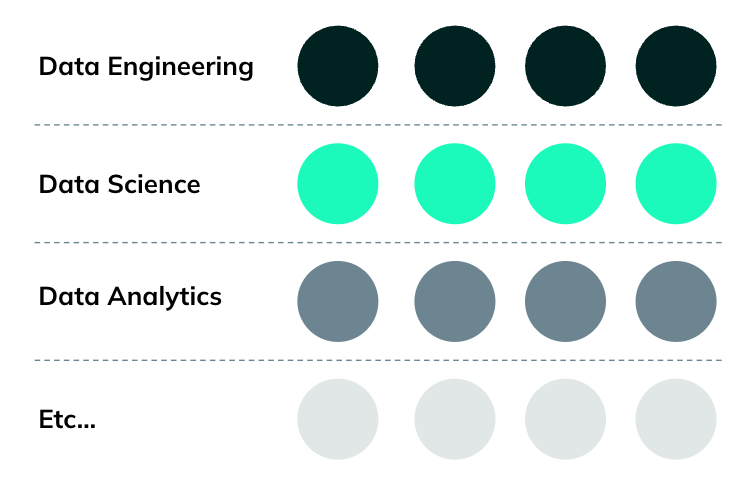
This is a fairly standard approach in companies. It creates expertise by skills, as each layer finds it easy to develop common practices and tools.
The drawback is that the technical stack resulting from this organization mimicked the different technological layers. Their interoperability was low and this showed whenever one project required crossing several of these layers.
The stack is the consequence of the organization (Conway's law). An organization by centers of expertise produces a technologically-layered stack.
This model gradually caused friction as business needs increasingly grew and the data team struggled to operate effectively at scale.
Before they knew it, the Data team had become a big bottleneck. Their delivery speed of large projects was slowing and the prioritization of work across technological layers was difficult.
Some topics, such as data quality, even had ambiguous owners. For example, the process of absorbing and delivering data from new entities became very long and complicated and as more Data Science use cases that required streaming data were appearing, the data architecture was too rigid to address these needs.
Faced with this growing problem, the need for organizational change became obvious.
Specifically, the Data team needed multidisciplinary squads tied to business domains so that they could operate effectively and autonomously to meet growing business needs and autonomously.
So What: What should BlaBlaCar look like?
As a data practitioner, I thought there was no better inspiration than the principles of Data Mesh.
A Data Mesh architecture is a decentralized methodology that empowers domain teams to independently conduct cross-domain data analysis. Central to this approach is the domain, which has its own dedicated team responsible for managing operational and analytical data. The domain team collects operational data and creates analytical data models, transforming them into data products for their own analysis. Additionally, they have the option to publish data products with data contracts, catering to the data requirements of other domains.
The theory may sound perfect, but will it work in practice? What else can we look for guidance on how to make it happen?
The benefit of Data Teams is that we can always look to the Product and Engineering teams next door for best practices. They have experienced similar challenges in the past and indeed, multidisciplinary feature teams have become common in Software Engineering. From speaking with the Product and Engineering teams at BlaBlaCar, we could validate the multidisciplinary approach and took notes on how to implement it.
How: How BlaBlaCar transformed the Data team
A change of this magnitude definitely doesn’t happen overnight. It is achieved by incremental changes with learnings and adjustments incorporated along the way. Specifically, this is what happened in BlaBlaCar’s Data department:
- Q3 2021: Diagnosis. Understand the problem that the department faces. With a clear problem statement, build a vision towards the solution.
- Q4 2021: Onboarding of managers to align on the problem statement and the target vision. If they do not own change, they will not lead it effectively.
- Q1 2022: Creation of 2 squads following the new principles, out of 6 new teams in the target vision.
- Q2 2022: After integrating feedback, creation of the remaining 4 teams.
- End of 2022: All reporting line changes made, and 2 squads are already in their 3rd quarter of operation.
- 2023: Focus on the technical migrations to complement the organizational changes. Change started with the organization, because success hinged on a mindset shift.
It may look simple when written like this but delivering the change brought up many critical and sometimes difficult decisions.
1. How to choose which teams to start with?
BlaBlaCar started with the easiest areas to make independent. We factored in known internal moves, which helped to accelerate some changes. The last teams to be created were those whose domains were more intertwined and harder to isolate.
2. Should we work on all aspects of the Data Mesh at the same time?
The answer is specific to each organization and its priorities.
Data Mesh is a paradigm that influences various aspects, including tools, stack, skills, organization, processes, and mindsets. While it encompasses all these dimensions, it is not necessary to excel in every aspect to achieve a functional implementation that suits your organization’s needs.
At BlaBlaCar, the Data As A Product aspect is perhaps the one where there has been the least investment at this stage of the transformation.
Typically, there is no Data Product Owner for each domain at Blablacar.
Likewise, the autonomy of squads to the point of managing their ingestion pipelines themselves has not been pushed that far. Data ingestion pipelines are still centralized. Decentralizing them added non-urgent changes for squads, while they already had substantial changes to absorb.
3. Are there any changes to be made outside of Data, especially on the Business side?
Again, the answer will depend on the context.
At BlaBlaCar, there was very little organizational impact outside of the data team because the change was designed to mirror the business organization. The Data department remained independent and by molding their organization into the major business areas, they managed to stick close to their stakeholders.
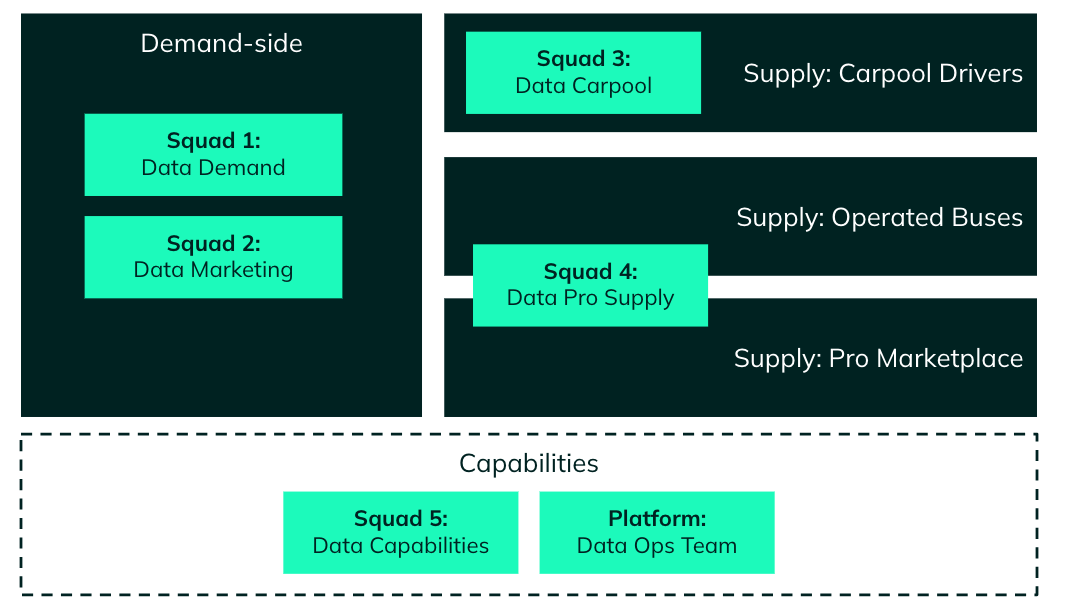
4. What about keeping the expertise and practices created in each area?
BlaBlaCar wanted to still keep and maintain common practices among the experts distributed in squads, so we created Chapters. These are vertical communities of practice by expertise where everyone can meet, challenge their technical choices and learn from their peers.
In fact, the Chapters embody the notion of federated governance. For instance, one or more members of each Chapter are part of the governance group. They guarantee the convergence of practices despite the dispersion of people within the different business squads.
Autonomy and boundaries between squads need a counterweight: federated governance and common practices.
From a people management point of view, it is also a way for BlaBlaCar to prevent managers from handling too many responsibilities. Having Chapter Leads is therefore also a way to empower individual contributors.
This governance task force manages subjects that affect both data governance (cataloging, quality, lineage, etc.) as well as team governance (operating rules and synergies between teams) or perimeters (moving borders, creating new domains).

5. How do we keep the Data machine well oiled?
BlaBlaCar maintains a horizontal data squad called Data Ops who provides the necessary infrastructure and services to the other squads - they keep the Data machine well oiled.
For instance, Data Ops builds ingestion patterns consumed by the Data Engineers in the squads .
The ingestion of production data is managed by the central team, but the transformations are the responsibility of the squads.
On a daily basis, the Data Ops team synchronizes with the Data Engineering Chapter, which represents all the squads. This makes it possible to speak with one voice regarding all potential constraints at the squad level. This one voice enables rapid convergence around problems, and thus, solutions.
In the implementation, particularly with regard to technical migrations, the Data Ops team prepares them, and communicates with the squads when they can carry them out.
With the current size of the data entity, this operation is tenable without this central team being a bottleneck. As the team grows and matures, some parts will likely be redistributed into squads.
To avoid creating silos, members from the DataOps team often temporarily join squads and vice versa in an exchange. This has two great benefits: guaranteeing that the tools and patterns provided by the Data Ops team meet the needs of the squads (who are their customers), and allowing team members to explore and learn other topics.
A few tips to conclude
This transformation was above all a human challenge. When performing such changes, don’t underestimate the time needed to align on the problem and solution. It is therefore not so much a technical challenge as a change management challenge.
If you are wondering if this is the right time for you to consider a transformation to Data Mesh, here are some tips that we’ve learned at BlaBlaCar:
- Making a Mesh organization if you only have one squad does not make sense.
- Below 3 to 4 squads, it is important to ask the question of whether it is really necessary.
- Beyond 3 to 4 squads and if there is a need for a great diversity of expertise within the squads, it starts to become interesting because we quickly arrive at an organization of 30-40 people.
The risk is decentralizing too early. This creates silos and divergent practices when your teams are not mature enough. It is therefore important to already have teams or communities of common practice upstream.
If there's one thing to remember, it's that you shouldn't try to implement a solution if you don't understand the problem you're trying to solve.
The Data Mesh is a solution, but it is important to make your own diagnosis before starting headfirst into it!
See also
More insights to better the world through technology