Is Data the New Holy Grail for Platforms?
The more people search online, the more data they generate, the more Google can improve their algo performance and personalise the search experience, making their engine in turn more valuable to each user. Pretty amazing uh?
This is called “data network effects (nfx)”. Within a data network, they theoretically occur when every node in the network feeds useful data back to the central database. As the aggregated data accretes, the value of the service provided to each individual node grows consequently. Think of Waze where users of the app feed back real time data (e.g. works signalisation, traffic bottlenecks etc.), hence increasing the utility of the app for the other users.
That being said, we previously stated that data network effects — although a powerful potential source of defensibility — typically tend to be weaker than many people often want to believe and might take time to materialise. Indeed, there are a couple of shortcomings to their beautiful theoretical existence.
Are data network effects a myth?
First, the value of incremental data typically goes down. At first, your machine learning algorithm learns very quickly, but you can quickly get to an asymptote, with new data being added to the model not translating into the same level of accuracy improvement. Second, the “cost of data” can go up significantly: it can gradually become more expensive & time consuming to collect useful data and to maintain the data infrastructure (i.e. growing computational costs, extensive data-points labelling, increasing security costs etc.). Third, often the relationship between product usage and the amount of useful new data gathered can be asymmetrical: on TripAdvisor, not everyone is a contributor; most users passively use the platform. And finally, data network effects are only one type of defensibility one can build with data: they are often confused with data advantages that can come from mere scale; data “embedding” can also ensure product stickiness through high switching costs.
In the end, can data really generate a strong moat? Are data nfx a sustainable source of competitive advantage for your company?
It appears that it could be the case under very specific preconditions defined below in a 7-points framework.
The Data-nfx 7 points-framework
- 1. The product/service value should increase with more incremental data.
Short and positive feedback loops should be built into the product to ensure that the new data generated is quickly incorporated back into the model. And product and/or services’ value increases automatically as more data is added.
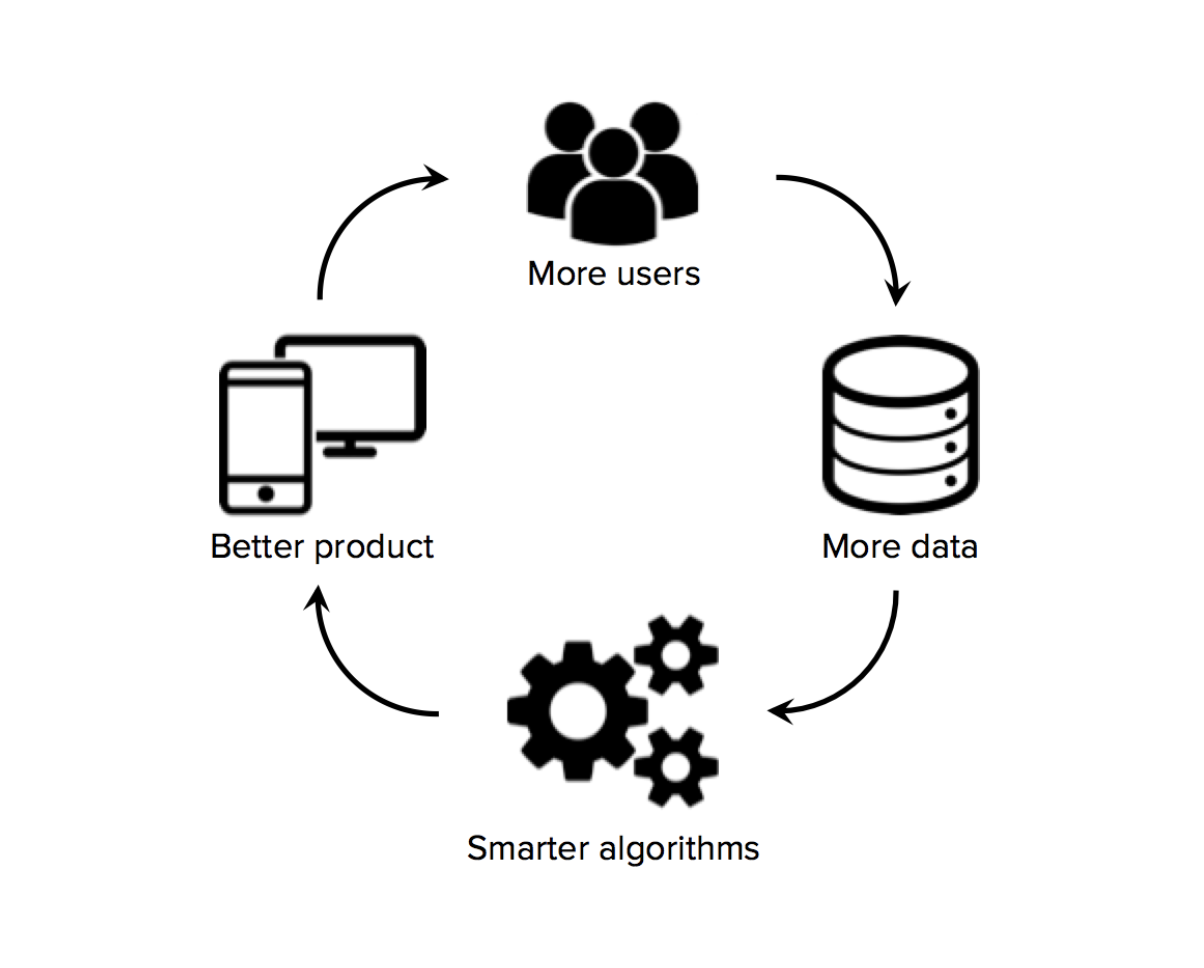
- 2. Higher product/service usage generates more useful data.
Ideally data production is native, with proprietary data generation (vs. inputing third party data into the model); and the new data produced is of high quality.
- 3. The value created by the data is core to the model’s value proposition & to the way the product/service benefits the user.
Data needs to be central to the way the product benefits its users. For instance, recommendation engines using data to push personalised content are typically only a feature of product or service and not its core: the data nfx of a Netflix or of a Spotify tend to be weaker than advertised. At the end of the day, the main value of such platforms lies in the content itself.
- 4. There is no asymptotic value of additional data.
This is typically the case if the service provided is real-time or dynamic for instance: highly dynamic datasets entail perpetual learning curves. It could be fine to have an asymptote, yet if it’s the case the threshold at which this asymptote occurs need to be super high.
- 5. There are no asymmetries in data contribution by members of the network.
All nodes need to contribute to the data network and produce useful data. For instance, the ML model behind Gboard is fed by anonymised typing data coming from all Android devices.
- 6. The data production/collection process is scalable and cost effective.
Ideally data capture and data labelling can be automated to keep the cost of new data production & incorporation low.
- 7. The “minimally viable corpus” (i.e. min. amount of data one required to start training the model) needs to be high.
The threshold for the amount of data required before the product starts providing value needs to be high as to provide scale defensibility against competitors entering the market. For instance, one of the key strengths of the research platform Owkin is their proprietary network of hospitals and clinics, providing them with large, real-world health datasets to fuel their disease detection models. This is very linked to the marketplace infamous chicken & egg challenge.
Final considerations
Under those 7 conditions, data network effects could potentially be an interesting source of defensibility. At Samaipata, we invest in digital platforms displaying increasing returns, and we believe that data nfx are becoming a possibility for a much broader group of companies, even at early stages. This is allowed by recent advances in technologies such as i) Big Data tools allowing for cheaper and faster infrastructure to process large amounts of data, ii) advances in Machine Learning / Deep learning and an increasing number of off-the-shelf tools and algorithms to automatically analyse and learn from those large amount of data, and finally iii) the rise of Cloud computing to be able to process it. The next generation of engineers increasingly moving from R&D-types of projects to business cases is also contributing to the market momentum as tech talent is fuelling into the startup scene.
**
At Samaipata, we are always looking for ways to improve. Do not hesitate to send us your thoughts. We strive to partner with early-stage founders and to support them in taking their business to the next level. Check out more ways in which we can help here or for all our other content here
And as always, if you’re a European digital business founder looking for Seed funding, please send us your deck here or subscribe to our Quarterly updates here.
See also
More insights to better the world through technology